Research Topic
Our goal is to connect our expertise in bioinformatics, computer science, and statistical genetics to better diagnosis and treatment of diseases.
As a part of the Department of Medicine, we have two main roles:
(1) We develop advanced methods and algorithms for genetic and genomic analysis, to enhance our understanding of the human genome.
(2) We extensively collaborate with clinical researchers to help design, analysis, and interpretation of the genomic analysis for clinical/translational research.
Roles (1) and (2) are interconnected; working closely with clinical researchers provides us a unique opportunity to identify unmet needs of new methods, and helps us to develop practical solutions that can be directly applied to clinics.
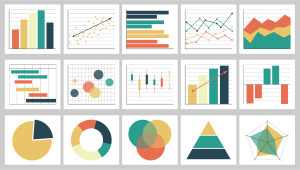
Statistical Methods for Genetic Association Studies
We make novel computational and statistical approaches to facilitate design, quality control, and analysis of genome-wide association studies.
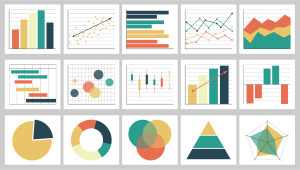
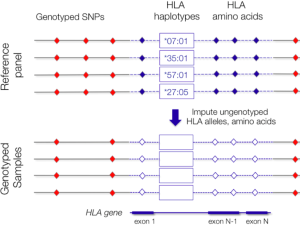
HLA Imputation & Fine-mapping
We make imputation approach for HLA region and fine-map MHC region which is a highly challenging region for genetic studies.
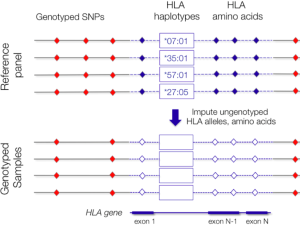
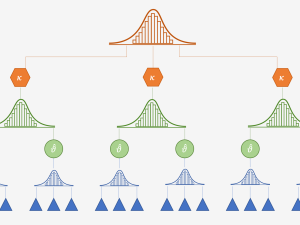
Meta-analysis
We develop new methods for meta-analysis that increases power. We expand the application of meta-analysis to eQTL analysis and GxE interaction detection.
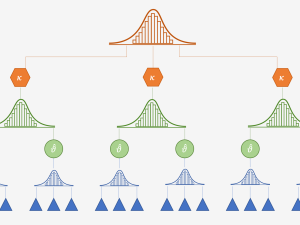
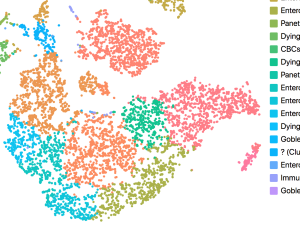
Single Cell Analysis
We develop new tools for analyzing single cell RNA-seq data, targeting better visualization, automatic cell type prediction, marker discovery, and clinical interpretation.
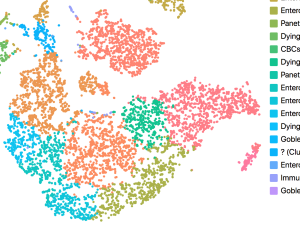
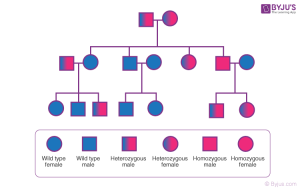
Heritability Analysis
We dissect and analyze the heritability of complex traits using advanced tools for genomics. We also develop methods that can obtain insights from diverse genomic architecture of diseases.
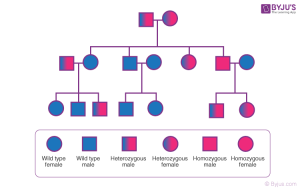
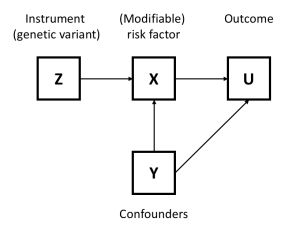
Mendelian Randomization
We are aiming at discovering novel causal relationships and verify assessing associations with clinical end points. Again, we develop several extensions to MR approaches.
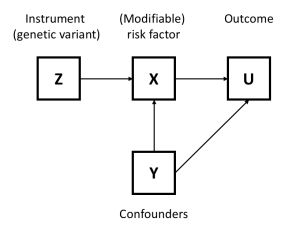
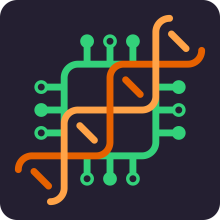
Privacy Protection & Cancer Genomics
We develop algorithms that can help privacy protection of individual data by applying novel encryption algorithms to genomic and medical data.
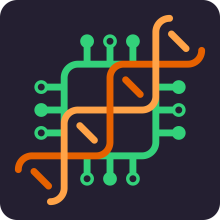
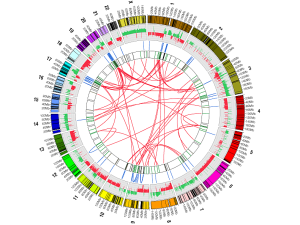
Multiomics Data Integration
We make systematic frameworks that can integrate different types of omics data, including NGS data, scRNA data, GWAS data, eQTL data, and microbiome data.
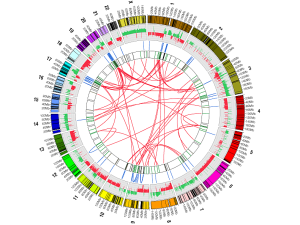